Going Vertical
The lines demarcating AI layers are blurring. We are increasingly seeing model providers move up the stack into the application layer. Where are we headed with this?
The news cycle might have been taken over by the tariff tantrums and the consequential market volatility, but the tech cycle continues to be dominated by the AI cycle’s progress. There was some big news coming out of the AI model providers this past week. All with a common thread or theme.
Exhibit A:
Google Cloud Next 2025 and the days following up to it where it made multiple announcements
Its enhanced GPU and TPU lineup including Ironwood, along with new Nvidia Blackwell GPUs on Google Cloud
Gemini Flash and Pro updates and its integration with their audio and video models like Chirp 3, Lyria and Veo 2
Vertex AI - Its Agent ecosystem in the Cloud, including the A2A protocol (for multi-agent systems to communicate with each other), with data, security and coding agents
On top of that Gemini and its AI integration within the Workspace environment
This full stack approach is now finally allowing Google to unify its presence across the infrastructure, model, orchestration and application layers to establish a coherent AI story. Bears labelled Google as not being nimble enough, too slow and too late to the AI models party and its core search business being under existential threat. The numbers do look quite lopsided for its biggest competitor in the model layer.
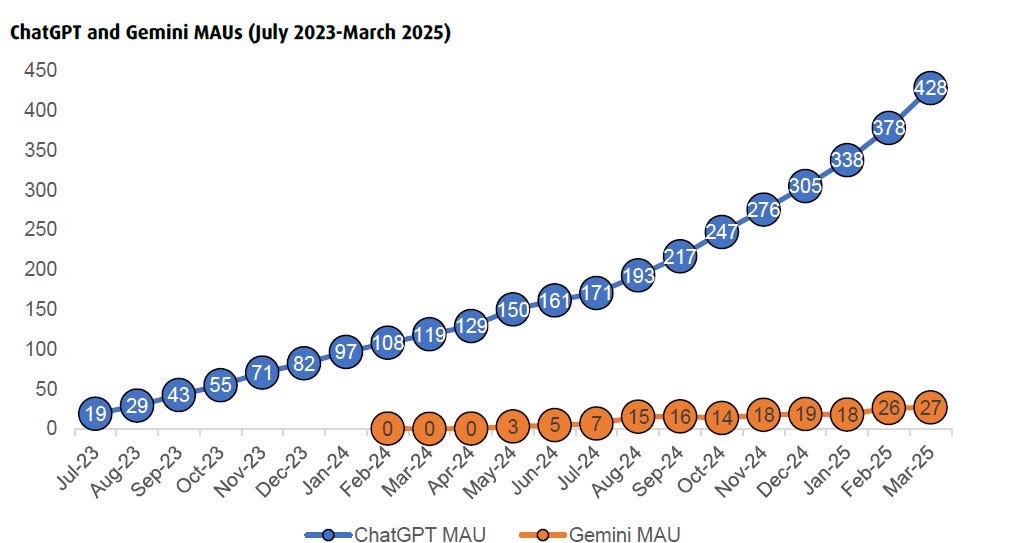
Could this vertical integration be an effective way to compete with much smaller players against their individual moats in each layer? After all it has the largest distribution moat, across various consumer and enterprise touchpoints (Search, Chrome, Android, Youtube, Workspace, Gmail and others).
All of this and we have not even factored in DeepMind and all the developments in their Alphafold series of models, or the progress on the Waymo front which is now at 250k autonomous robotaxi paid rides per week.
Exhibit B:
Open AI was in the news (no surprise there) for few different things. The most noteworthy of them was the Bloomberg leak on its discussions to buy Windsurf, an AI coding assistant for $3B, which was the valuation at which it was trying to raise its latest round.
AI coding assistants has been one of the hottest application layer areas that has been attracting capital left, right and centre from investors. Microsoft made that move early by buying out Github, which launched its Github Copilot last year. Others like Replit, Anysphere (Cursor), Magic, Supermaven, Poolside and Lovable have continued to raise fast (at >25x ARR in a lot of cases) at multi-billion dollar valuations.
Instead of just powering these assistants with an API, Open AI has now made a move upwards to enter the application layer itself. Jamin Ball had a nice writeup last week on it and some of the implications of AI labs moving ‘up the stack’.
The model cost curves have continued to fall from a cliff as inference price of LLMs have decreased between 9x and 900x since 2021 decreasing more than 10x every year (depending on the tasks).
As the commoditization of AI models and APIs continues, moving into “products” seems like a natural move for the model providers to maximise revenues and profits.
According to reports, Open AI is also working on its own social network trying to build an image generation social app . Seems ludicrous, but it is again another bite out of the application layer by owning an AI social app.
More importantly, it lacks what Meta and X have, the vast treasure trove of real-time user generated Instagram, Facebook, Threads and X posts that continuously feed and improve the Llama and Grok models. Something that is extremely valuable in the race for marginal improvements in frontier models, amongst other use cases.
Remember it also has a GPT App Store?
Exhibit C:
Anthropic, another leading AI Model lab released its own coding assistant, Claude Code powered by its latest Sonnet 3.7 model as it saw Claude was the preferred model for developers.
Then, it released its own AI application communication protocol (the MCP) which in its own words is like a USB-C port for AI apps, providing more context to LLMs connected to apps.
Last week it alos launched its Research assistant as well which is able to integrate with Google Workspace and search across both the web and internal work.
While it has not forayed deep into the application layer directly yet, the intent is clear. Extend your competitive advantage by providing tools across the stack.
AI is going vertical
We might no longer be able to look at model providers confined to their own territory as they begin to vertically integrate upwards. A few examples of this in previous tech cycles give us a good look into how this fared in the past:
The IBM Mainframe era where it designed and manufactured hardware (mainframes like System/360), developed the operating systems and core software, provided maintenance and services, and handled sales and leasing directly. They controlled almost the entire value chain for their customers.
Apple in the Mobile / PC era where it designs its hardware (Mac, iPhone, iPad), develops its own operating systems (macOS, iOS), designs its own core processors (Apple Silicon), controls the primary software distribution channel (App Store), operates its own retail stores, and offers integrated services (iCloud, Apple Music, etc.). While it outsources manufacturing, it maintains tight control over design, components, and the overall ecosystem.
In a way Neflix in the Streaming era could be similar where it began as a distribution platform (DVDs, then streaming licensed content). It strategically integrated backwards into content creation, becoming a major producer of "Netflix Originals." It now controls both the global distribution platform and a significant library of owned content.
Does it make sense though for this cycle?
Janelle Teng used Clayton Christensen’s Modularity Theory pretty well to answer the question in her recent piece.
In markets which are not fully mature enough (where a performance gap exists), competitive advantage goes to interdependent builds and vertically integrated companies since customers value functionality and reliability at this point.
Whereas in markets which have a performance surplus, competitive advantage goes to modularization through specialization, outsourcing, and dis-integration, since this is where other factors beyond core functionality, such as speed, responsiveness, and convenience, tends to win.
AI is still not in the mature bracket yet that needs modular architectures, and where products are not good enough, so a full stack approach or building a moat in multiple layers could stillyield significant benefits. Its all about building durable competitive advantages at this point, but it may change as we move along this cycle.
It could reshape the current ecosystem
With the model providers moving into the application layer, and incumbents like Google trying to compete across the stack, they are directly competing with smaller players in the application layer they are serving. This could reshape the current dynamics as one might wonder if the APIs powering the third party apps will have access to the best models as the one driving their own apps or tools?
Akin to IBM’s own software and database systems competing with third party software providers in the mainframe era, Microsoft’s tightly coupled OS and Applications competing with independent PC application providers in the PC era, or Apple’s own apps and tightly controlled ecosystem competing with third party apps in the Mobile era.
We are slowly leaving the “operator neutral infrastructure” territory.
Counterintuitive, but capital deployment in the Application layer might continue to trend upwards
Application layer AI native companies have attracted a ton of capital, which in 2024 was $8.2B, up 110% YoY. These companies are also growing much faster than their previous generation SaaS companies, with the AI cohort reaching $1M in revenue at a median of 11 months as compared to the latter at 15 months.
Model layer companies and other “integrated” giants are deep pocketed and more established than most of their smaller application layer counterparts. Quite naturally, investors would look at them now entering the space where they are deploying capital and start discounting the diminishing returns on the marginal dollar invested given increasing competition with integrated players.
However, it may be the case that now application layers start looking like attractive acquisition targets for model providers and internet giants, which might push more capital towards AI apps as a new exit avenue opens up for investors.
The Davids vs the Goliaths
The Davids, the smaller AI application builders might be wondering where to go next as incumbents have started to enter their space early in the cycle and offer the “good enough features”.
Taking a page out of Christensen’s Disruptive Innovation theory in this story, they might have to focus more on simplicity and ease of use, focusing on customer niches that are small or too specialised for the large incumbents. They might also add more value if they can quickly become the best tool for a specific user in their vertical, iterating faster than larger players can.
The cycle has taken an interesting turn where moves made by AI labs and incumbents are showing signs of benefits of vertical integration outweighing the integration, market disruption and regulatory risks.
But what competitive advantages might sustain going forward in this AI cycle? And in what layers?
Until next time,
The Atomic Investor