We have got to Build it, but will they come?
A massive capex cycle to build out the AI infra. is making investors wary due to demand uncertainty. Drawing some parallels with the last cycle shows that all hope might not be lost just yet.
AI has been the only talk in town for some time now. Its share of mind continues to go up as it has an increasingly larger presence at dinner table conversations, networking events, company boardroom meetings, and other group chats.
Both public and private markets seem to be relaying this sentiment as well. Stocks viewed as AI beneficiaries have been on a tear making them the only idea that seems to be working at the moment.
The S&P 500 gained 33% since October 2023 until June 2024, and 56% of those gains have been linked to AI beneficiaries - Nvidia, other semiconductor stocks, and Big Tech (Apple, Amazon, Microsoft, Google, Meta).
The gains have been even more concentrated since Q1 this year, with Nvidia, other semis and Big tech as a group up 45%, 17% and 11% respectively (until June 2024), while everyone else as a whole is…down 2%.
In Venture land, AI/ML deal values and valuations continue to climb, with Gen AI infrastructure and horizontal platforms (like model builders which cut across industries and use cases) attracting most of the capital.
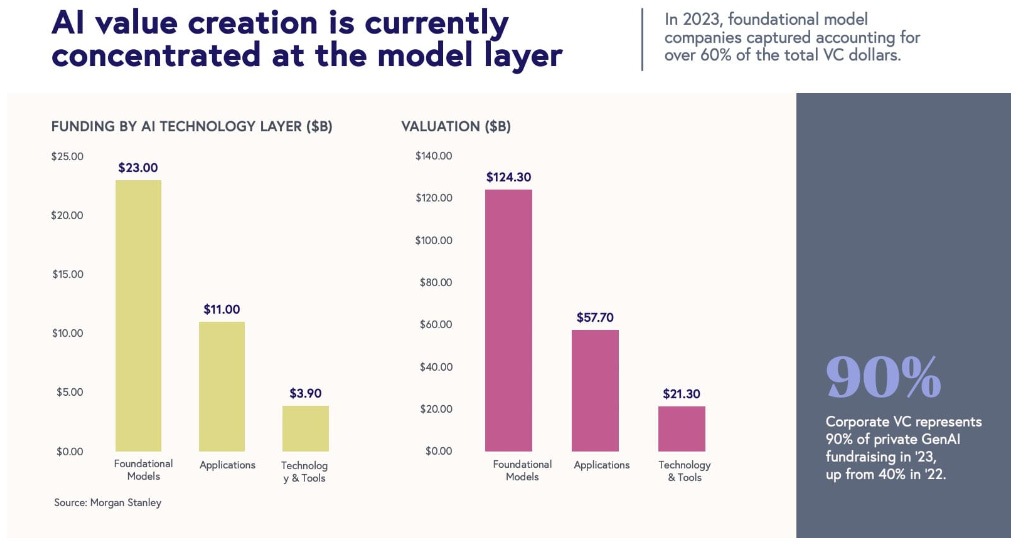
These concentrated gains in just one area of the market are far from reflecting the true economic reality, and seem to be driven mostly by excitement, momentum and FOMO.
Given that estimates of future revenues and profits have not moved much, a lot of the gains have come at the expense of rising valuations which implies pricing in even higher expectations of AI delivering substantial value for investors, businesses and consumers going forward.
Underinvesting is a bigger risk
The AI cycle is not being played out in vacuum. The ChatGPT moment last year kickstarted a massive capex cycle as companies realised they needed to build out the underlying AI models and the compute infrastructure to suffice a surge in demand for AI use cases.
AI needs an enormous amount of compute resources (processing power). For reference, vs a simple Google Search, ChatGPT requires 10x the amount of compute, while a video generation model requires 10,000x of it.
This is translating into several hundred billions of $$ worth of GPUs, servers and data centre build outs needed to serve the AI infrastructure layer. Even a16z has decided to hoard some Nvidia GPUs (20,000 of them according to media coverage) to attract startups building in the space.
Big Tech, which also includes the 3 largest hyperscale Cloud providers is at the front and centre of the AI arms race, building out their own models (both proprietary and open source) and providing AI compute and Cloud-based tools for the rest of the world to build their AI services on top.
Given the breadth and depth of use cases of AI and GenAI and pace at which it is evolving, both consumers and enterprise demand has exceeded the existing compute capacity needed to serve it.
“The demand for all of these AI products and services is so high right now that we are just doing crazy things to make sure we've got enough compute to fulfill the demand.” - Kevin Scott - Microsoft CTO
Analysts are estimating a total of $1tn of AI related capex till 2030, an unprecedented ramp up in spending for a technology cycle. The YoY % change in capex tells you all where this is headed.
We have fast tracked to a point where not investing is a bigger risk given the lead time it takes to build out capacity, train the models and get them ready for inferencing projects. There is no turning back from the AI arms race, something that was echoed in the Q2 earnings calls this past week as well.
“It's hard to predict how this will trend multiple generations into the future, but at this point, I'd rather risk building capacity before it is needed rather than too late, given the long lead times for spinning up new inference projects” - Mark Zuckerberg (Meta CEO)
“When you go through a curve like this, risk of underinvesting is dramatically greater than risk of overinvesting” - Sunder Pichai (Alphabet CEO)
“The reality right now is that while we're investing a significant amount in the AI space and in infrastructure, we would like to have more capacity than we already have today. I mean we have a lot of demand right now. And I think it's going to be a very, very large business for us” - Andy Jassy (Amazon CEO)
Data centre buildouts are then leading to second and third order capex cycles - upgrading existing energy infrastructure and building out new grids to serve the additional power demand of these AI data centres, expected to push the overall power demand considerably.
“By 2030, the power needs of these data centers will match the current total consumption of Portugal, Greece, and the Netherlands combined.” - Goldman Sachs Research
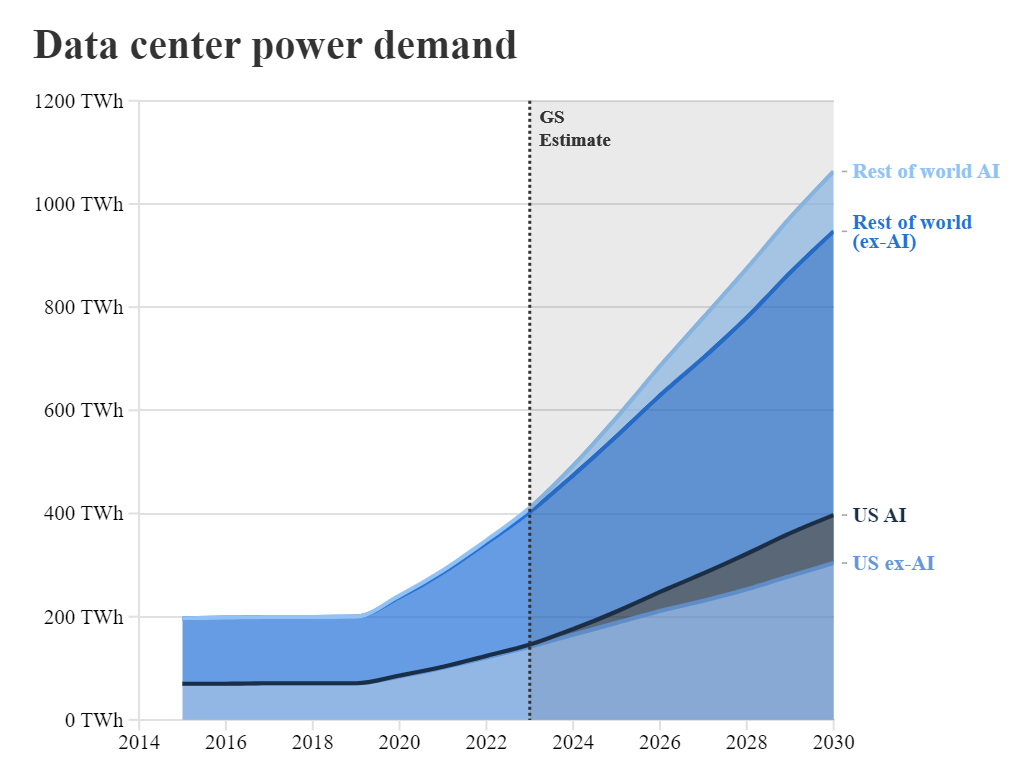
Europe alone needs a $800bn to upgrade the old power grids to support AI data centres, while US utilities will need to invest billions to support higher power demands due to AI compute. Add $800bn or so on top for renewable energy capacity and you have $2tn of additional capex just to support the AI infrastructure being built out.
A classic example of supply scarcity combined with a pull forward in demand, eventually leading to overinvesting and possible oversupply in the future.
We have got to build it. However…
The economics of AI are not easy to digest
Sequioa came out with an interesting piece recently trying to estimate the required revenue for the payback on AI capex spend, opining that there is a $500bn revenue hole which keeps getting bigger as the AI spend creeps up (even after assuming the largest tech companies have $10bn of AI-related revenue).
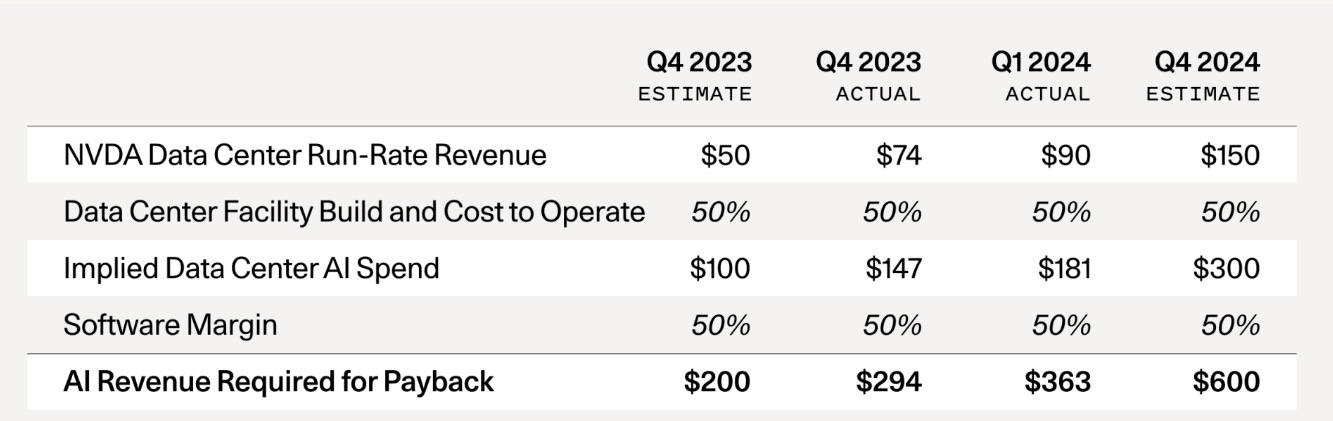
Investors are starting to question by when and if even companies would be able to convert these invested dollars into revenues and cash flows as the current market structure and economics are not geared towards attractive returns. Notable hedge fund Elliot Management has already come out recently saying that AI is overhyped and Nvidia is a bubble.
Models are getting more expensive to train as they get larger. GPT-4 took $78mn to train, Google’s latest Gemini Ultra $191mn, while Meta’s state of the art Llama 3.1 $640mn.
“The amount of compute needed to train Llama 4 will likely be almost 10x more than what we used to train Llama 3. And future models will continue to grow beyond that” - Mark Zuckerberg (Meta CEO)
These are exponential increases in fixed costs with not much clarity on if those extra training costs are worth the marginal performance gain (and for what use cases or applications).
Then API pricing (cost to use those models) is already extremely competitive. Most independent model providers are losing money at this stage according to analysts.
Additionally, increasingly less differentiation with the one of the best models now being open source is likely to make it even harder to compete in the application layer, with everyone having access to the same (or similar) models. Commoditisation of AI products and services means low pricing power and barriers to entry where most of your profits get competed away.
The Application layer is not having an easy time either. In the decade of the Cloud, it had north of 70% Gross margins, but AI apps today have sub 50% gross margins.
Another risk that is less often discussed is how quickly the value of your equity can depreciate as every new model or GPU comes out and your capital is stuck in the last gen products or technologies which are now worth a lot less.
Big Tech seems to be well positioned to capture a lot of the value as their core businesses and products get better with AI, with almost all of their capital intensive R&D and capex projects funded with existing cash flows. But how much more are the additional future cash flows worth?
The current setup maybe has some parallels with another cycle in the past.
We might have been here before
A $500bn revenue hole with not so good looking economics has put investors in a tough spot, and the markets are already starting to feel jittery.
Can we look at a previous comparable tech cycle to find some parallels to try to get some comfort with what is going on in the current one?
The Dot Com cycle of the 1990’s, the Internet era, saw massive investments in building out the connectivity infrastructure. Telcos and Cable companies like AT&T, Verizon, Cisco, Juniper, Sun and others spent $500bn in laying down new lines, installing switches and routers and also building out wireless networks.
Telco capex went from $47bn in 1995 to $121bn in 2000. Many small and large firms like Global Crossing and Worldcom went bankrupt as they could not service the debt they had used to fund the network buildout.
“One reason for the massive increase in the capacity of the long-haul network was bullish expectations for future demand. For instance, a CEO of Level 3 Communications, a large long-haul firm, stated that Internet traffic would double every three to four months. That didn’t happen, and estimates are that demand (in terms of volume of data, not in terms of dollars spent) may be doubling every year, which is a significant increase but is substantially shy of expectations.” - The Boom and Bust in Information Technology Investment
VCs also invested billions of dollars into companies with a lot of hype and unsustainable, unprofitable business plans as VC investment reached $98bn in 2000 from just $18bn two years earlier. A lot of capital was incinerated as many seemingly early winners of the cycle like Pets.com, UrbanFetch, WebVan and other dot coms went bust.
“At the beginning of 2000, there were between 7,000 and 10,000 substantially funded internet companies. Three years later, 5,000 of those companies had either been acquired or had closed their doors. The capital destruction amounted to trillions of dollars.” - Embrace the GenAI mania
The key here is that overinvestment led by expected demand growth for bandwidth created a glut and oversupply in just a few years leading to a price erosion for bandwidth. It has suffered massive declines every year since.
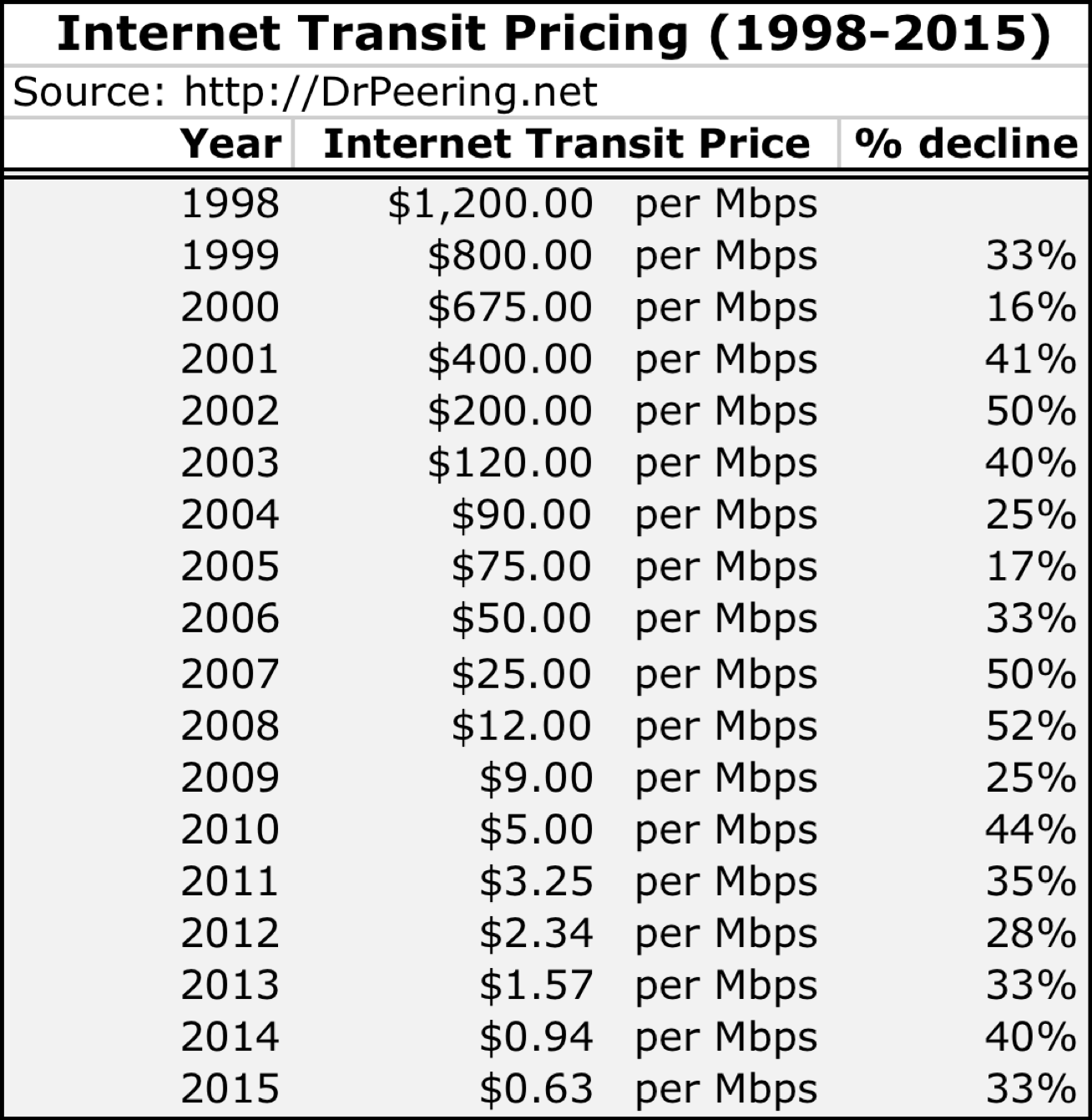
The infrastructure and service providers in the Internet era HAD to build the internet backbone, overestimating demand in the short run but maybe underestimating in the long run.
A capex cycle like this one therefore is likely to last for years. Scarcity leading overinvestment, turning into a glut which then leads to price declines is something we have witnessed before in the 1990s.
The comparable today would be inference costs which are priced as cost/million tokens (a token is a chunk of text the LLM generates or processes). Inference prices have already started to come down considerably.
“when Mistral released its Mixtral LLM in December, a flurry of inference startups began offering it at increasingly lower prices, and its price dropped from around $2 per million tokens to just 27 cents in a matter of days”
Companies like Character.AI, which was recently “Acquihired” by Google have reduced inference costs by a factor of 33x since 2022.
Then there are efficiencies in the compute intensity and data centres led by products like the Nvidia GB200 which is 25x more energy efficient than the previous gen chip. So the capex intensity and costs might further fall driven by demand fluctuations and more efficient hardware design and software.
On the adoption side, the 90s saw PC hardware and mobile handset companies (the enablers) like HP, Dell, Microsoft, Intel, Nokia etc. allowed people to get access to the Internet and increase adoption, basically starting from near zero.
ChatGPT allowed AI to break into the mainstream last year and we have both upstarts and incumbents giving people access to AI on a massive existing install base of PCs, Smartphones, Internet, Cloud and Applications.
Adoption today has gained good momentum for both enterprises and consumers, and companies are willing to invest in the space to experiment if they can create more value for their business and customers.
This might further be fuelled upwards by declining costs and oversupply going forward, which could then allow AI to penetrate smaller enterprises and individuals providing them an economically viable platform to build on top of.
There are concerns galore, like every other technology cycle when it is still unclear if there will be enough value created and who the eventual winners might be (if any). Incumbents seem to be capturing most of the value, but just like the last cycle, new winners in specific niches and verticals might emerge that are not out there at the moment.
What we are also likely to witness is multiple corrections in the market along the way, with a lot of capital at risk being burnt and a few home runs that pull more even more capital in. Its almost like a feature and not a bug for every major technology epoch in history.
No cycle plays out exactly like the last one. But if the cost and the adoption curves continue on similar trajectories, and we can find ways for AI to deliver actual consumer and economic value, all hope might not be lost just yet.
Until next time,
The Atomic Investor